Problem: Find potential acetylcholinesterase
inhibitors (AChEI) in a commercial screening compound database. |
Strategy:
Develop a profile for acetylcholinesterase inhibitors by looking for other
activities that are important for this class of compounds.
Overlap in multidimensional activity space predicted compounds and
compounds with experimentally known activities. |
Hypothesis:
Compounds that are in the same region in the activity space have a very high
probability of having similar biological activities.
|
Experiment (1):
We select all acetylcholinesterase inhibitors from the principal list in MDDR.
Principal list means only those compounds are selected that are the
principal compounds in a patent or report.
We predict the PASS parameters with pa>80% for all these compounds. We
find a limited number (18) of other activities that are important for some
of these compounds.
We use the set of 18 activities to screen in silico the OAK
Samples database (OAK).
|
We combine the compounds from MDDR and all compounds with a PA>50% from
OAK in an Excel spreadsheet. (If you want, you
can download (0.8 MB) the zipped spreadsheet following this link. The
spreadsheet contains the structures and was produced with ISIS
for EXCEL.)
We display the data using miner3d.excel, plotting first the activities
"acetylcholinesterase inhibitor" against "cognition
disorder"
|
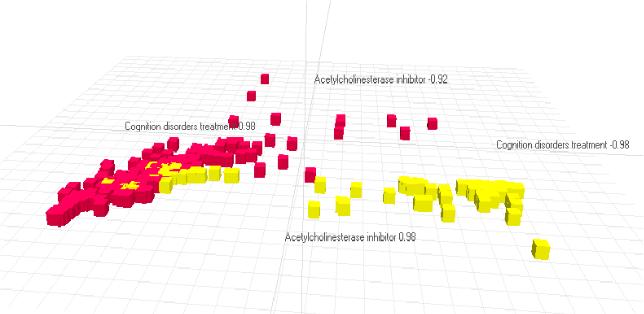 |
and we see a nice
clustering of the red (MDDR) and the yellow (OAK) points |
The next pictures shows the points plotted in three dimensions,
"acetylcholinesterase inhibitor", "cognition disorder" and
"cholinergic agonist". |
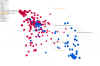 |
The final picture shows as fourth activity "miotic". The points
were filtered so that more points with similar values for all activities
become visible (balls). |
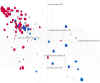 |
The following table shows a few selected compounds, displayed as balls in
the previous plot. |
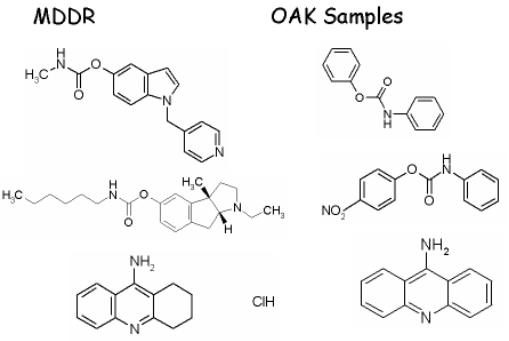
|
Experimemt (2):
The same as in experiment (1), but we use a set of activities that might be
relevant for actylcholinesterase inhibitors. (We thank Prof. U. Jordis from the
Techncal University in Vienna for his suggestions.)
We used in addition to MDDR and OAK Samples, a set of compounds from the NCI
database that had pa > 0.7. Download
the EXCEL spreadsheet with the calculated PASS parameters, and try for
yourself analysing the data using miner3d.excel
|
We created the plot AChE vs. cognition disorders treatments vs cholinergic
agonist from Experiment (1) and the plot AChE vs. ACh nicotinic agonist vs. ACh
nicotinic antagonist from Experiment (2) structures. form both plots, we select
a cluster of compounds that overlapped with the structures of MDDR. This is
fairly arbitrary way of selecting compounds, and in spite of this we found a
large overlap among the selected compounds. This encourages us that or
hypothesis, see above works. You can download
here the EXCEL spreadsheet with the results. |
Plotting the activities:
AChE
ACh nicotinic agonist
ACh nicotinic antagonist
cognition disorders treatments
cholinergic agonist
we selected the following compounds to to be active as acetylcholinesterase inhibitors:
|
You need the Chime add-in to see the structures |
|
|
Experimemt (3):
An experienced chemist looking at the results of experiment 2, the compounds
that are selected as potential AChE inhibitors, recognizes that these
substances are not very drug like. Most of the compounds are flat, missing
chiral centers and are polyaromatic compounds. Instead of using the limited OAK
Samples database, we could use ACD-SC. This is a
database of over 1.5 million compounds containing structures of most of the
suppliers offering screening compounds.
This database is very large for desktop
machines and was not installed on our system. The chance of finding drug like
compounds would have been much larger.
|
However, we can try to find a real
candidate using a database
like MDDR that contains only drug like compounds.
We searched for "Acetylcholinesterase Inhibitor" in the principal
list of 27554 compounds and found 141 hits.
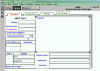
|
We subtracted these from the principal list, exported the
SDFile, generated
the PASS parameters, and selected all compounds with Pa-Pi > 0.3. We generated
a spreadsheet containing these compounds, the 141 experimentally known AChE
inhibitors and as comparison a list of compounds from the commercially available
Maybridge database. You can download the zipped file mddr_pricipal-ache_maybridge.zip. We looked for compounds who overlapped with the known AChE inhibitors in the
biological space consisting of AChE inhibitor, cognition disorder treatment,
antiparkinsonian and cholinergic agonist.
|
We selected a few compounds and looked
at them again in MDDR. We found one compound that was not classified as AChE
inhibitor in the "Activity" field of MDDR, but was tested active as
AChE inhibitor, according to the description in the "Biology" field.
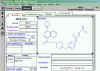
|
This is the text in the section Biological Activity in MDDR:
ACTION - Neuroprotectant and cerebral function improving agent, acetylcholinesterase inhibitor (IC50=3.7
mcM); compound may be useful in the treatment of Alzheimer's disease and other memory disorders. Other
compounds from a series of aminonaphthalene derivatives are the
|
following:183158 183159 183160 183161 183162 183163.
We believe this is a very convincing result showing the ability of PASS and
miner3d.excel to be helpful in identifying new promising AChE inhibitors. |
In the above experiment, we also included compounds from the Maybridge
database with Pa-Pi > 0.5. None of the compounds overlapped with known AChE
inhibitors in the biological space consisting of AChE inhibitor (AChEI) and
cognition disorder (CogD) treatment. However, we found another application of
using the biological spaces. We only looked at the Maybridge compounds and
plotted these data in other biological spaces.
|
We found some clustering using a
5 dimensional space using ACheI, CogD, cholinergic agonist, antiparkinsonian,
and adenosine A1 receptor agonist. A chemist selecting screening compounds would
select a few representatives from each cluster. In close comparison to the use
of chemical diversity when selecting screening samples, a chemist can select now
compounds using "biological diversity". |